A new way for estimating the total mass of clusters of galaxies from Planck microwave images with Artificial Intelligence techniques
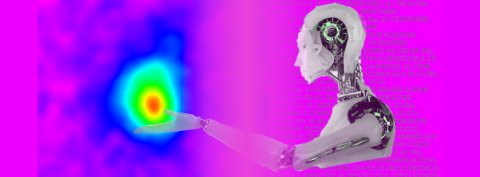
Galaxy clusters are the largest gravitationally bound structures that have ever formed in our Universe. They are increasingly used as useful laboratories to study the evolution of the Universe and the accurate measurement of their total mass allows us to constrain relevant cosmological parameters.
However, estimating the mass from multi-wavelength observations and with different physical assumptions and models, lacks accuracy and/or precision due to unavoidable systematic errors.
Among the various classical approaches, a Machine Learning model has been applied for the first time onto images of the Sunyaev-Zel'dovich (SZ) effect signal, provided by the Planck satellite, to infer the true total mass of galaxy clusters reliably and accurately.
Machine learning (ML) is a powerful domain which uses Artificial Intelligence to make predictions with data. A lot of applications of ML have appeared in many different fields of industry and research in recent years. It not only accelerates the process of efficiently dealing with a great amount of data, but also brings new methods and new findings. An international group of researchers, including experts in galaxy cluster science from Universidad Autonoma de Madrid (Spain), University of Edinburgh (UK), MIT (USA), Sapienza University (Italy) and experts in ML models from EURA NOVA company (Belgium), have started a collaboration to provide new ways to deduce the mass of galaxy clusters from multi-wavelength images.
Recently, they have focused on accurate estimates of the mass of galaxy clusters using microwave images from the Planck satellite.
The basic idea proposed by this group is to overcome all physical assumptions to infer the cluster mass by different observables and to get the total mass directly starting only from observed images. The ML model is based on one type of Deep Learning algorithm, the Convolutional Neural Networks (CNN), which can link the most important features present in an image to a defined quantity: the total mass. The approach is powerful only after a well training phase. This procedure has been performed with almost 200 thousand images of the SZ signal of synthetic galaxy clusters extracted from the hydrodynamical simulation of The Three Hundred project.
The inferred cluster masses result close to the true mass and with a very small scatter. The subsequent application of this model to the images of the Planck satellite, provided unbiased mass of over 1000 galaxy clusters, differently from the observational assumptions in Planck.
This study has been published in the latest Nature Astronomy issue.
A natural extension of this ML application in cluster science has been a recent project for a Master Thesis in Astronomy and Astrophysics. The fully integrated radial profiles of gas and total mass in clusters were recovered starting from SZ mock images. A paper on this subject has been submitted to MNRAS.
Authors at the Department of Physics, Sapienza University of Rome:
Marco De Petris, Antonio Ferragamo, Giulia Gianfagna and Alessia Sbriglio.
References:
Nature Astronomy paper https://www.nature.com/articles/s41550-022-01784-y
MNRAS submitted paper https://arxiv.org/abs/2207.12337
Info:
Marco De Petris
marco.depetris@roma1.infn.it